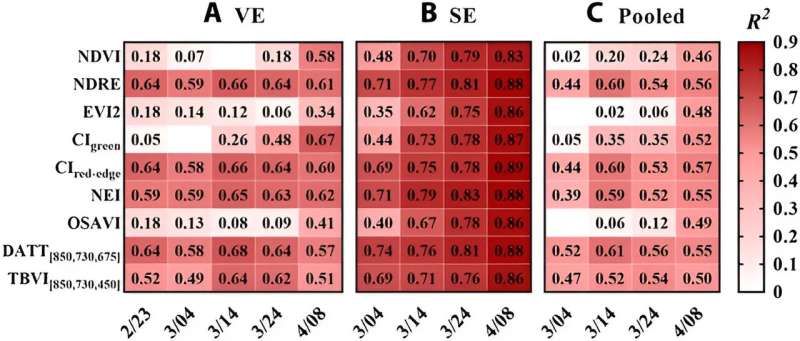
Wheat is crucial for global food security, and panicle number per unit ground area (PNPA) is key to its yield. Traditional manual counting methods are accurate but inefficient, prompting a shift towards remote sensing and image processing for rapid, nondestructive PNPA estimation.
Recent studies have primarily used near-ground platforms for accurate, small-scale PNPA estimates, but their efficiency is limited. Unmanned aerial vehicles (UAVs) offer a promising alternative, especially pre-heading, using multispectral imagery to manage and increase yields effectively.
However, challenges remain, such as spectral saturation affecting accuracy and the need for improved methods integrating spectral and textural analysis to overcome this. Additionally, the impact of exposed background materials on these estimates is not fully understood, and further research is needed to refine PNPA estimation techniques for wheat.
Plant Phenomics published a research article titled “SPSI: A Novel Composite Index for Estimating Panicle Number in Winter Wheat before Heading from UAV Multispectral Imagery.” This study introduced a spectral-textural panicle number per unit ground area (PNPA) sensitive index (SPSI) derived from unmanned aerial vehicle (UAV) multispectral imagery to improve PNPA estimation in winter wheat before heading by mitigating spectral saturation.
The SPSI combined an optimal spectral index (SI) and textural index (TI) to address the effects of background materials on PNPA estimates. The performance of SPSI was compared with traditional SIs and TIs, revealing that green-pixel TIs generally outperformed all-pixel TIs, with specific exceptions. SPSI demonstrated superior overall accuracies and significantly reduced spectral saturation compared to other indices.
Moreover, it showed improvements in correlation coefficients and reductions in root mean square error and relative root mean square error when applied to two experimental datasets.
The relationships between PNPA and various indices were examined, revealing that specific SIs exhibited stronger relationships with PNPA. The texture-based indices derived from green pixels exhibited significant differences in performance, with green-pixel-based TIs generally providing higher correlation coefficients.
The research identified sensitive bands for constructing SPSI, noting the consistent and overlapping bands across different dates. This led to identifying two COR-based normalized difference texture indices (NDTICORs) that were particularly sensitive to PNPA before booting. SPSI, combining DATT[850,730,675]and NDTICOR[850,730], was closely associated with PNPA, inheriting the advantages of both indices and demonstrating less sensitivity to cultivation factors.
The study further investigated the sensitivity of SPSI to various spectral uniformity scenarios and cultivation factors, indicating its robustness and lower sensitivity compared to DATT[850,730,675].
Modeling and validation results consistently affirmed the superior performance of SPSIs in PNPA estimation across different datasets, particularly around the optimal timing of estimation. In conclusion, the research concluded that incorporating textural information into a composite index effectively mitigates spectral saturation and improves PNPA estimation, offering potential benefits for crop yield prediction and precision agriculture, especially when applied to high-resolution satellite imagery.
More information:
Yapeng Wu et al, SPSI: A Novel Composite Index for Estimating Panicle Number in Winter Wheat before Heading from UAV Multispectral Imagery, Plant Phenomics (2023). DOI: 10.34133/plantphenomics.0087
Citation:
Revolutionizing wheat yield prediction: Introducing SPSI for enhanced panicle number estimation using UAV imagery (2023, December 29)
retrieved 31 December 2023
from https://phys.org/news/2023-12-revolutionizing-wheat-yield-spsi-panicle.html
This document is subject to copyright. Apart from any fair dealing for the purpose of private study or research, no
part may be reproduced without the written permission. The content is provided for information purposes only.