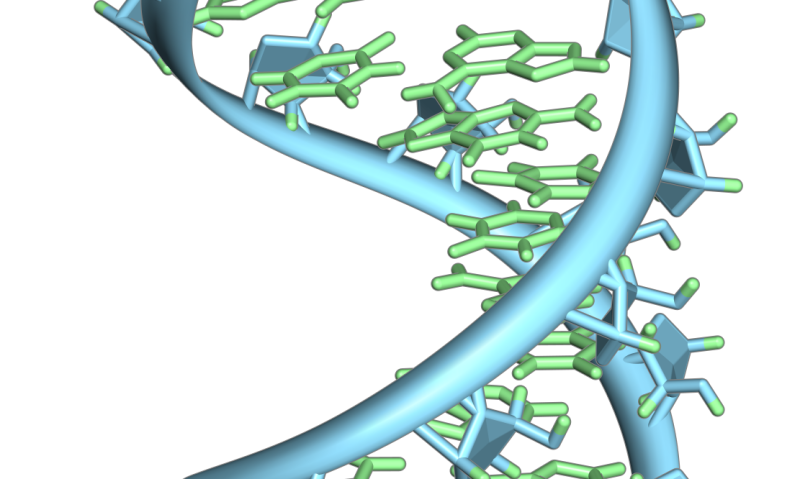
A new statistical technique developed by a researcher at the Texas A&M University School of Public Health and colleagues elsewhere offers fresh insights into how diseases affect individual cells. This innovative method, known as hybrid Bayesian inference, blends different statistical approaches to better understand complex diseases like idiopathic pulmonary fibrosis, which has long puzzled scientists due to its elusive nature.
The technique was developed over the past decade by Gang Han, Ph.D., and others for use when prior information is available for some parameters but not others. This approach paves the way for more precise medical discoveries in many areas.
“This hybrid approach is better than the frequentist inference because it includes prior information and also better than the Bayesian inference because it reduces the problem of bias resulting from noninformative priors, which can be significant with small sample sizes,” said Han, who is a professor at the School of Public Health.
The current single-cell RNA sequencing technology faces the same challenge when it comes to identifying the genes of interest. Differentially expressed genes can be identified by pooling single-cell RNA sequencing into the same biological replicates, but the small sample size reduces their power. On the other hand, acquiring large sample sizes can hardly be possible due to high cost and prolonged accrual of patients with certain diseases.
For their current study in Human Genomics, Han and colleagues from the pharmaceutical company Eli Lilly and Company applied the Bayesian-frequentist hybrid framework to a case study involving idiopathic pulmonary fibrosis. Using a semisynthetic data source of single-cell RNA sequencing of mouse hypothalamus, the researchers studied the statistical power and false discovery rate of the Bayesian frequentist hybrid inference compared to other analysis methods.
They then used all three methods—the Bayesian frequentist hybrid inference, frequentist and Bayesian methods—to analyze gene expression data from a lung tissue dataset. Specifically, they studied the association between idiopathic pulmonary fibrosis and transforming growth factor beta 1, adjusting the probability of cells being alveolar macrophage cells.
The researchers found that the Bayesian frequentist hybrid inference identified more genes of interest as long as there was a proper informative prior and that these genes were reasonable based on the current knowledge of idiopathic pulmonary fibrosis. When frequentist inference and Bayesian inference were used, either no differentially expressed genes, or only a small number, were found.
“Our most unusual finding was that our method can trigger full-on pathway analysis for the identification of genes associated with disease status of a specific cell type,” Han said. “This means that it can identify significant genes in a specific type of cell that are relevant to a particular disease given limited sample size.”
This study builds on a 2022 paper on the computation of hybrid inference. In that study, Han and other colleagues applied the Bayesian frequentist hybrid inference to a biomechanical engineering knee implant design and a study of the relationship between the location and type of acral lentiginous melanoma—a form of skin cancer—and the selected surgical approach for treating it. In both cases, the approximation outperformed both frequentist and Bayesian inferences with sample sizes ranging from 20 to 500.
Next, Han plans to conduct studies on multivariable hybrid Bayesian analysis that incorporate more information on patient demographics into the model and has other sources of information as environmental variables.
“The bottom line is that Bayesian frequentist hybrid inference not only adds valuable insight into idiopathic pulmonary fibrosis, but also is a unique and flexible framework for many other future single-cell RNA sequencing analyses,” Han said.
More information:
Gang Han et al, Bayesian-frequentist hybrid inference framework for single cell RNA-seq analyses, Human Genomics (2024). DOI: 10.1186/s40246-024-00638-0
Citation:
Innovative statistical method reveals new insights into single-cell RNA sequencing (2024, September 22)
retrieved 23 September 2024
from https://phys.org/news/2024-09-statistical-method-reveals-insights-cell.html
This document is subject to copyright. Apart from any fair dealing for the purpose of private study or research, no
part may be reproduced without the written permission. The content is provided for information purposes only.