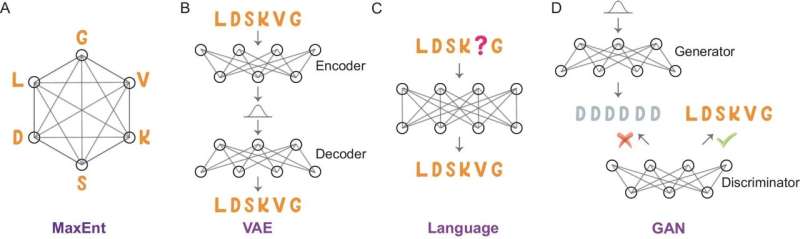
Enzymes, nature’s remarkable biocatalysts, play an integral role in various aspects of daily life. Consider the awe-inspiring sight of fireflies lighting up a summer night. Their captivating glow serves not just to enchant observers but also plays a role in communication and mating. This natural spectacle is powered by an enzyme known as luciferase.
Zooming into the molecular scale, enzymes are intricate networks of amino acids. Luciferase, a prime example, exhibits a fascinating diversity among different species. However, these homologs exhibit specific evolutionary patterns, crucial for advancing the prediction of protein structures. A key question emerges: how do these patterns relate to the function of enzymes?
Dr. Xie and Dr. Warshel aim to decipher this intricate relationship. They approach proteins as a language of amino acid ‘letters’ and are developing generative AI tools akin to the principles behind the widely used ChatGPT.
Their AI tool quantifies the likelihood of certain sequences or mutations appearing in nature. Fascinatingly, they discovered that this probability is primarily linked to enzyme catalytic activity when mutations occur near the substrate. Conversely, mutations in the enzyme’s scaffold mainly correlate with protein stability. These insights have enabled the successful engineering of several proteins, including luciferase.
Dr. Warshel said, “The relationship between evolutionary information and diverse enzyme functions suggests that enzymes are indeed multi-scale objects. This study has the potential to revolutionize our understanding of enzyme catalysis and evolution.”
This research, now published in the journal National Science Review, not only offers new perspectives on enzyme catalysis but also holds promise for practical applications in biotechnology and beyond.
More information:
Wen Jun Xie et al, Harnessing generative AI to decode enzyme catalysis and evolution for enhanced engineering, National Science Review (2023). DOI: 10.1093/nsr/nwad331
Citation:
Generative AI illuminates enzyme secrets using sequences evolved in nature (2024, March 20)
retrieved 20 March 2024
from https://phys.org/news/2024-03-generative-ai-illuminates-enzyme-secrets.html
This document is subject to copyright. Apart from any fair dealing for the purpose of private study or research, no
part may be reproduced without the written permission. The content is provided for information purposes only.